The Past, Present & Future of Statistics in the Era of AI: Speaker Details
Browse speaker bios and abstracts from the GW Department of Statistics' 90th anniversary event, "The Past, Present and Future of Statistics in the Era of AI."
Visit the event page for full details.
PLENARY SPEAKERS
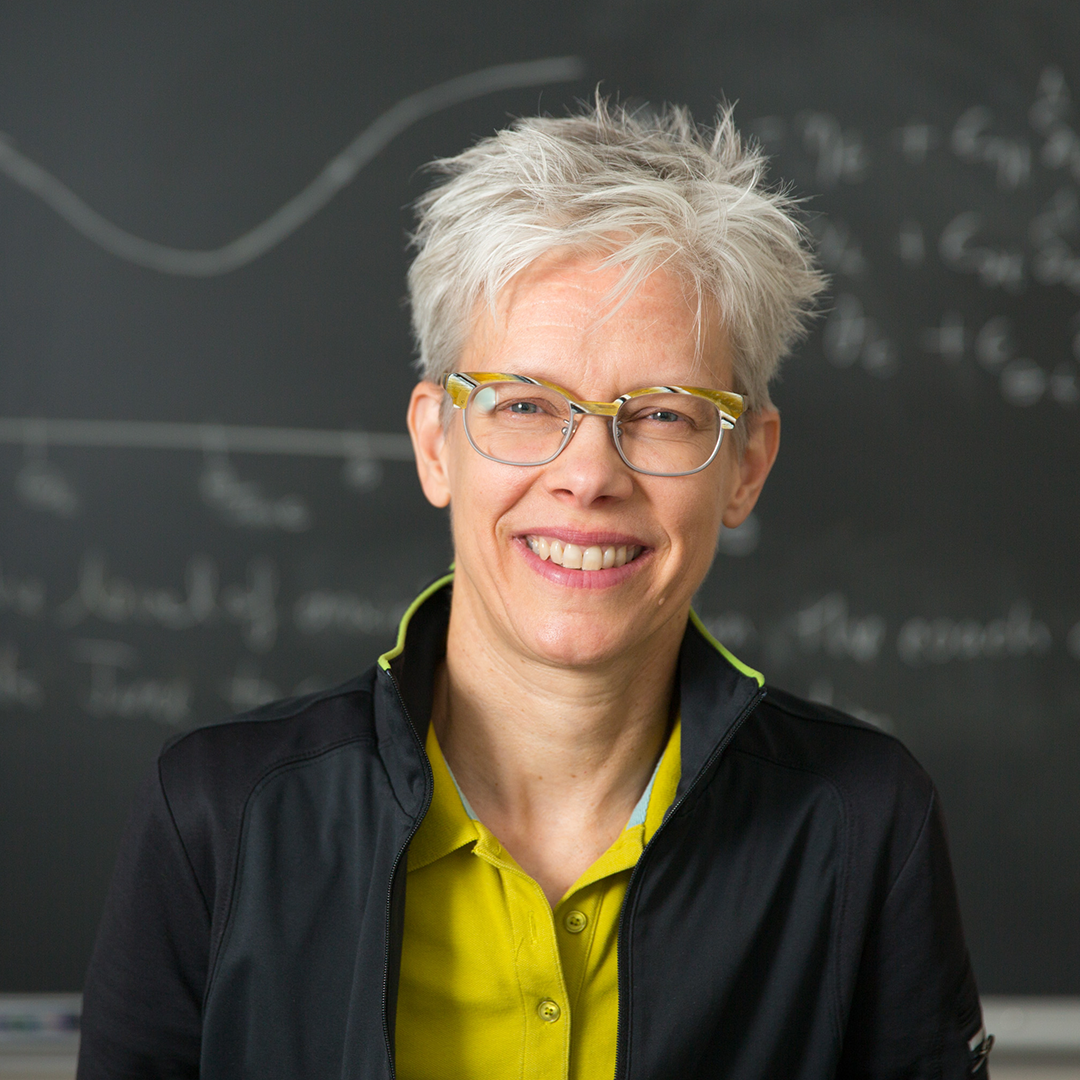
Susan Murphy
Mallinckrodt Professor of Statistics & of Computer Science
Associate Faculty, Kempner Institute | Harvard University
"Online Reinforcement Learning in Digital Health Interventions"
In this talk I will discuss first solutions to some of the challenges we face in developing online RL algorithms for use in digital health interventions targeting patients struggling with health problems such as substance misuse, hypertension and bone marrow transplantation. Digital health raises a number of challenges to the RL community including different sets of actions, each set intended to impact patients over a different time scale; the need to learn both within an implementation and between implementations of the RL algorithm; noisy environments and a lack of mechanistic models. In all of these settings the online line algorithm must be stable and autonomous. Despite these challenges, RL, with careful initialization, with careful management of bias/variance tradeoff and by close collaboration with health scientists can be successful. We can make an impact!
- About Susan Murphy
Susan A. Murphy is Mallinckrodt Professor of Statistics and of Computer Science and Associate Faculty at the Kempner Institute, Harvard University. Her research focuses on improving sequential decision making via the development of online, real-time reinforcement learning algorithms. Her lab is involved in multiple deployments of these algorithms in digital health. She is a member of the US National Academy of Sciences and of the US National Academy of Medicine. In 2013 she was awarded a MacArthur Fellowship for her work on experimental designs to inform sequential decision making. She is a Fellow of the College on Problems in Drug Dependence, Past-President of Institute of Mathematical Statistics, Past-President of the Bernoulli Society and a former editor of the Annals of Statistics. Visit Susan Murphy’s website.
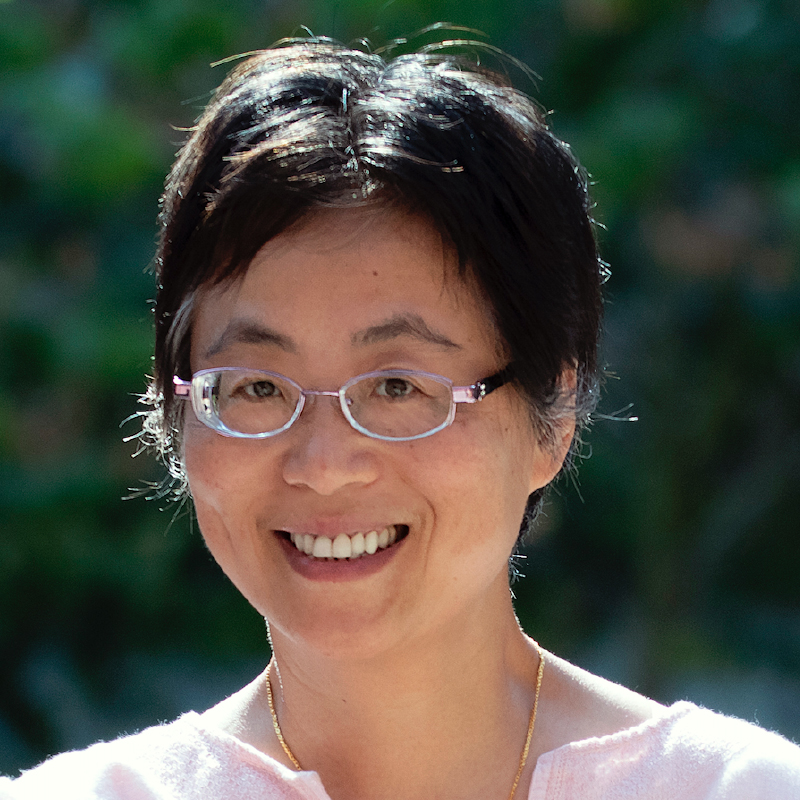
Annie Qu
Chancellor’s Professor
University of California, Irvine | Department of Statistics
"Representation Retrieval Learning for Heterogeneous Data Integration"
In this presentation, I will showcase advanced statistical machine learning techniques and tools designed for the seamless integration of information from multi-source datasets. These datasets may originate from various sources, encompass distinct studies with different variables, and exhibit unique dependent structures. One of the greatest challenges in investigating research findings is the systematic heterogeneity across individuals, which could significantly undermine the power of existing machine learning methods to identify the underlying true signals. This talk will investigate the advantages and drawbacks of current data integration methods such as multi-task learning, optimal transport, missing data imputations, matrix completions and transfer learning. Additionally, we will introduce a new representation retriever learning aimed at mapping heterogeneous observed data to a latent space, facilitating the extraction of shared information and knowledge, and disentanglement of source-specific information and knowledge. The key idea is to project heterogeneous raw observations to the representation retriever library, and the novelty of our method is that we can retrieve partial representations from the library for a target study. The main advantages of the proposed method are that it can increase statistical power through borrowing partially shared representation retrievers from multiple sources of data. This approach ultimately allows one to extract information from heterogeneous data sources and transfer generalizable knowledge beyond observed data and enhance the accuracy of prediction and statistical inference.
- About Annie Qu
Annie Qu is Chancellor’s Professor, Department of Statistics, University of California, Irvine. She received her Ph.D. in Statistics from the Pennsylvania State University in 1998. Qu’s research focuses on solving fundamental issues regarding structured and unstructured large-scale data and developing cutting-edge statistical methods and theory in machine learning and algorithms for personalized medicine, text mining, recommender systems, medical imaging data, and network data analyses for complex heterogeneous data. The newly developed methods can extract essential and relevant information from large volumes of intensively collected data, such as mobile health data. Before joining UC Irvine, Dr. Qu was a Data Science Founder Professor of Statistics and the Director of the Illinois Statistics Office at the University of Illinois at Urbana-Champaign. She was awarded the Brad and Karen Smith Professorial Scholar by the College of LAS at UIUC and was a recipient of the NSF Career award from 2004 to 2009. She is a Fellow of the Institute of Mathematical Statistics (IMS), the American Statistical Association, and the American Association for the Advancement of Science. She is also a recipient of IMS Medallion Award and Lecturer in 2024, and Jon Wellner Lecturer in 2024. She serves as Journal of the American Statistical Association Theory and Methods Co-Editor from 2023 to 2025, IMS Program Secretary from 2021 to 2027 and ASA Council of Sections of Governing Board Chair in 2025. Visit the Qu Lab website.
ALUMNI LECTURERS
Kimberly Sellers
Department Head and Professor
North Carolina State University | Department of Statistics
- "GW Statistics: A Nod to the Past, Present, and Future"
The Statistics Department at the George Washington University has a rich history, and continues to produce fine talent. Our discipline is changing and advancing at a rapid pace. What can the Statistics Department do to continue the rich tradition over the next 90 years? This talk will recognize the past, present, and future of statistics with a particular focus to the GW Statistics Department and its contributions to the field.
William Rosenberger
Distinguished University Professor
George Mason University | Department of Statistics
- "Fisher and Randomization"
R. A. Fisher was a devoted Darwinian, and, like Darwin, created science out of nothing. The list is long, but one thinks of likelihood-based estimation, analysis of variance, principles of experimental design, and randomization as standing the tests of time. Such accomplishments ``from scratch” (or nearly so) can amaze the fine statisticians who made meaningful incremental contributions to work begun by others, the few ``greats” among us who invented something important, and the unusually perceptive introductory statistics student, alike. Fisher thought of randomization in the context of agricultural experiments, but it has impacted most profoundly the science of medicine. Bradford Hill brought randomization to clinical trials. The concept of randomization-based inference, now resurrected in causal inference, was largely forgotten as design and analysis became segregated, perhaps due to analysis software packages.
Susan Ellenberg
Emerita Professor of Biostatistics | Medical Ethics and Health Policy
University of Pennsylvania
- "Bioethics and Biostatistics: Irrevocably Intertwined"
Anyone involved in medical experimentation on human beings (or animals, for that matter) will inevitably face ethical dilemmas. Regulatory and funding agency requirements, and ethical guidelines for human research, leave room for debate on the approach to specific research efforts. In this presentation I will discuss some of the most contentious ethical issues I have had to wrestle with during my time at the NIH, the FDA and U Penn.
INVITED SPEAKERS
Jian Huang | The Hong Kong Polytechnic University
- "Leveraging Large Models for Statistical Analysis"
In this talk, we consider the problem of leveraging pretrained large models for statistical analysis in the context of generative learning. We first introduce two generative learning models: generative adversarial networks and continuous normalizing flows. We then use two examples to illustrate generative modeling with the help of a pretrained large model. In the first example, we propose a protein sequence generation approach based on data representations learned through a pretrained large protein data model. In the second example, we introduce a conditional flow model for image generation leveraging a pretrained large image model.
Yang Liu | Remnin University, China | GW PhD '22 (Statistics)
- "The Impact of Unobserved Covariates on Covariate-Adaptive Randomized Experiments"
Covariate-adaptive randomization (CAR) procedures are widely used in randomized control studies to achieve balance in observed covariates, thereby improving statistical efficiency and validity. Despite their success, two fundamental questions remain affecting the application of these methods: (1) Does balancing observed covariates also improve balance in unobserved covariates? (2) Is such balance sufficient to ensure valid inference of treatment effect? In this talk, I will present our recent work addressing these questions in the context of CAR with discrete covariates. Regarding the first question, I will introduce a theoretical framework for evaluating unobserved covariates imbalance. Our findings show that balancing observed covariates often leads to improved balance in unobserved ones, providing theoretical support for the advantage of CAR over complete randomization in balancing unobserved covariates. For the second question, I will examine the inference of treatment effect under a linear outcome model. We show that when unobserved covariates interact with treatment, the treatment effect may become non-identifiable, leading to inconsistent estimation. Even when no interaction exists, standard model-based inference procedures may exhibit reduced Type I error rates. I will further discuss robust adjustment methods that restore valid inference under CAR. These findings offer both theoretical insight and practical guidance for the better application of CAR.
Kannan Natarajan | Pfizer
- "Statistical Leadership in Advancing Innovation in Drug Development"
With the growing challenges associated with need for new pharmaceutical medicines for patients with unmet medical needs, statistical leadership plays an important role in transforming the traditional paradigm of drug discovery and development. Accordingly, statisticians and data scientists are now spearheading innovation across the drug development continuum, with notable impact and influence. In this talk we will highlight the significance of statistical leadership, with special reference to important developments, including use of Bayesian methods, advanced data analytics and technology, use of multiple data sources, and the criticality of enhancing collaboration among stakeholders.
Weijie Su | University of Pennsylvania
- "Do Large Language Models Need Statistical Foundations?"
In this talk, we advocate for the development of statistical foundations for large language models (LLMs) and explain why such an endeavor is both necessary and achievable. We present two fundamental characteristics of LLMs that necessitate statistical frameworks: their probabilistic, autoregressive nature in next-token prediction, and their complex, non-unique architectures whose underlying mechanisms remain largely opaque. We identify key areas where statistical foundations could advance LLM development, including uncertainty quantification, calibration, watermarking, evaluation, data mixture optimization, fairness, and privacy. We illustrate our argument through examples demonstrating how statistical insights can help develop frameworks for LLM watermarking and fairness alignment.
Lee J. Wei | Harvard University
- "Lost in Translation: Can AI Help?"
Over the years, the process of designing, monitoring, and analyzing clinical studies for evaluating new treatments has gradually adopted a fixed pattern. Clinical trialists have sometimes been slow to embrace new methodologies—possibly due to concerns about potential delays in the review process for drug approval or manuscript submission. This cautious approach to innovation in drug development stands in stark contrast to other technology-driven fields. Scientific investigation is an evolving process, and insights gained from previous studies about methodological shortcomings should guide us in planning and analyzing future trials. Unfortunately, inefficient or inappropriate procedures continue to be used, even when superior alternatives exist. In this talk, we will explore various methodological challenges and potential solutions. The objective of a clinical study is to obtain robust, clinically interpretable estimates of treatment effects from a risk-benefit perspective at the individual patient level, using efficient and reliable quantitative methods. We will discuss how to achieve this goal, drawing from real trial examples. This talk will engage with the concept of "translational statistics and data science.”
Zhuoran Yang | Yale University
- "In-Context Linear Regression Demystified"
We study how multi-head softmax attention models are trained to perform in-context learning on linear data. Through extensive empirical experiments and rigorous theoretical analysis, we demystify the emergence of elegant attention patterns: a diagonal and homogeneous pattern in the key-query (KQ) weights, and a last-entry-only and zero-sum pattern in the output-value (OV) weights. Remarkably, these patterns consistently appear from gradient-based training starting from random initialization. Our analysis reveals that such emergent structures enable multi-head attention to approximately implement a debiased gradient descent predictor -- one that outperforms single-head attention and nearly achieves Bayesian optimality up to proportional factor. Furthermore, compared to linear transformers, the softmax attention readily generalizes to sequences longer than those seen during training. We also extend our study to scenarios with non-isotropic covariates and multi-task linear regression. In the former, multi-head attention learns to implement a form of pre-conditioned gradient descent. In the latter, we uncover an intriguing regime where the interplay between head number and task number triggers a superposition phenomenon that efficiently resolves multi-task in-context learning. Our results reveal that in-context learning ability emerges from the trained transformer as an aggregated effect of its architecture and the underlying data distribution, paving the way for deeper understanding and broader applications of in-context learning.
WiFi Connection
- GWGuest, available to all visitors. Connect to GWGuest.
- Eduroam, available to GW community members with a User ID. Connect to Eduroam.